Background
To date, Dr. Sachan has significantly impacted technological sustainability by developing systems based on eXplainable AI (XAI) and blockchain. These innovation systems promote transparent decision-making and facilitate decentralized collaboration among financial institutions.
It has achieved some impact on mitigating social biases and the commercialization of new technologies in the financial sector in compliance with the regulations. In the future, the real-world deployment of Dr. Sachan's research is expected to generate promising measurable societal impacts.
Research
Households with lower wealth often face difficulties in securing housing loans or funding to start small businesses, as these endeavors require significant collateral from conventional lending sources such as retail banks. Individuals facing challenges in securing loans seek guidance by consulting an advisor or automated Artificial Intelligence (AI) systems to evaluate their chances of success before approaching lending institutions. Underserved individuals are frequently subjected to discriminatory biases influenced by their gender, ethnicity, and societal position.
The extent of this inequitable treatment is further magnified by their notable underrepresentation in AI training datasets, highlighting a significant gap in the system's inclusivity and fairness.
The underpinning research integrates blockchain technology with an explainable AI algorithm to develop a trustworthy AI advisory and transparent decision-making system to address the following challenges:
a) Decentralized data sharing mechanism: In this research, a blockchain-based decentralized data-sharing approach is designed to enable collaboration among various financial experts by different financial institutions. This approach enhances data-sharing incentives by addressing concerns associated with personal identity protection and centralized data access control. The collaborative approach enriches the data for developing an automated decision support system and expands the collective knowledge involved in processing loans for underserved communities. I have recently tested the first phase of this decentralized data-sharing technique and shared the findings at an IEEE Blockchain conference and extended work.
b) Explainable AI for high-stake decisions: Most high-performance AI algorithms, such as Deep-Neural-Networks are inherently black-box in nature and lack transparency in decisions. Stakeholders in financial institutions prioritize explainability in AI decisions for critical situations involving human lives or significant assets despite the availability of high-performance AI algorithms. In this research, explainable AI algorithms: Hierarchical Belief-Rule-Base and Maximum likelihood Evidential Reasoning are developed to provide transparent decisions. These methods are published in journal papers, and the refinement of these algorithms is under development.
c) Defence against adversarial attack: Centralized AI solutions are vulnerable to adversarial attacks, wherein malicious actors manipulate the algorithm's environment. This manipulation could involve adjusting the model's parameters and inserting distorted or misleading data samples. Such assaults can lead to incorrect decisions that not only result in considerable financial losses but also inflict substantial damage on the lives of underserved individuals and can potentially lead to serious legal issues.
This research employs blockchain technology to automate the auditing of AI algorithms. It leverages the immutability characteristic of blockchain, which enables prompt detection of tampering or attacks on data and AI systems. The initial test results are under review in a journal, and further improvement of this technique is under development
Impact
1. Mitigating Bias and Enhancing Transparency in Financial Decision-Making
The research at the University of Liverpool Management School (ULMS) has promoted the reduction of societal AI biases and trustworthy financial decisions for underserved communities. This impact was realized through a partnership with the Open Trellis Program supported by RVA Works Enterprise Support, Inc. (https://opentrellis.org/). This organization provides pro bono services, aiding underserved individuals in obtaining business loans.
The underpinned research conceptualized explainable AI and blockchain decision-support systems to provide unbiased and transparent lending decisions. Recent public testimony in Forbes highlights the research impact on loan accessibility: "Our efforts in credit risk optimization, blockchain, and explainable AI (XAI) are helping to expand the accessibility of small-business loans." Until now, the RVA Work has helped over 250 entrepreneurs who fall under median income and members of racial minorities such as African Americans and Latino Americans.
We conducted another research on AI-enabled auditing methodology to detect biased decisions by finance professionals and leakage judgment errors in AI algorithms. The formulated auditing technique was validated through the annotation of test results by four underwriters at Together Financial Services. This research is under review; the company's anonymity is maintained in the publication (Testimony can be obtained in the future).
Previous research is linked with regarding credit data and the associated company. It is cited in the report on Tech and the Future of UK Foreign Policy. It attests to the likelihood that the existing research and forthcoming contributions will continue to impact the governance and application of AI in finance.
2. Commercialization of Blockchain and AI in FinTech and LawTech
The research has raised awareness of the potential commercialization of integrated blockchain and explainable AI systems to design innovative products and services to support the needs of a broader demographic in the rapidly evolving technological environment. The study provides compelling applications in the Finance and Law sectors.
a. The real-world implications of this work have received prestigious recognition by the Forbes Business Council as the number one 'hot' trend for the potential significant impact on small businesses. The CEO of Open Trellis wrote a public testimony of our work to corroborate the received acclaim.
b. Local awareness of the research is achieved by a case study on blockchain and AI in the Whitecap Consulting Report for Liverpool.
c. Forbes Acknowledgment on Commercialization of Technology: "Dr. Swati Sachan has been advancing research ... bridging the gap between researchers and those commercializing the technology."
Future Measurable Impact
The future impact of AI decision-making systems can be quantified by monitoring the number of minority beneficiaries. The system's efficiency can be assessed by comparing the duration of loan approval across different demographic groups and by analyzing loan approval rates to identify potential discrepancies or biases. A qualitative perspective can be gathered by collecting user testimonials or case studies on how multiple technologies integration enhanced loan accessibility. I plan to secure KTPs and other research grants to achieve these impacts.
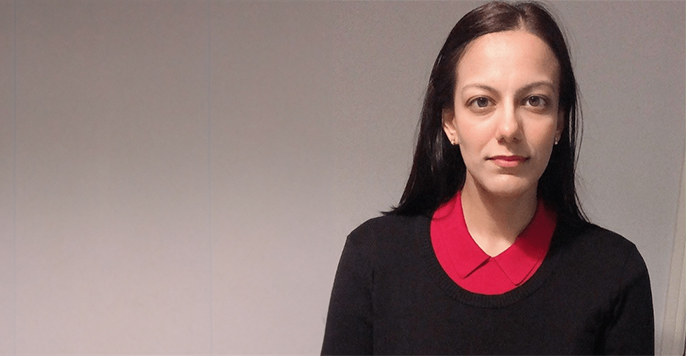
Dr Swati Sachan
Back to: Management School