New tools to measure real-time systemic risk and financial uncertainty
Posted on: 12 September 2024 in Research
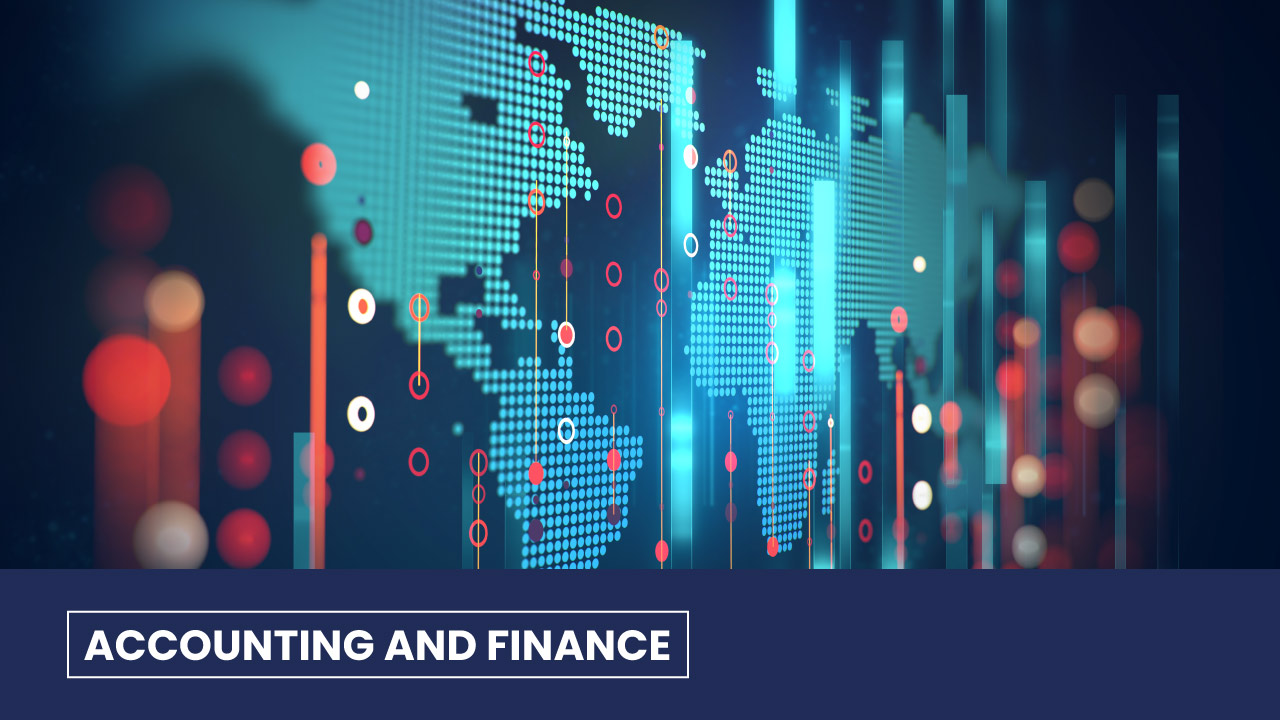
Based on a new model developed by Dr Michael Ellington that views links between businesses and sectors as a network, Dr Mattia Bevilacqua shows how we can detect industries with the greatest impact on the real economy.
Michael and Mattia propose and develop monitoring tools to identify sources of risk and financial uncertainty in real-time, with clear benefits for macroprudential supervisors and investors.
Michael proposes a framework that measures the strength, direction and persistence of links among businesses, and shows how connections among firms intensify during periods of high uncertainty and economic turmoil.
Using this approach, Mattia tracks connections using novel forward-looking data that provides a classification of “uncertainty hubs”, which are industries that amplify uncertainty to other sectors, impacting the overall economic cycle.
As well as showing how links among firms contribute to systemic risk, the models offer valuable insights for the design of policies, to prevent risk spillovers from the financial market into the real economy.
The impact of firm connectedness in financial networks
Prior to 2008, US banks embraced an aggressive form of predatory lending, targeting low-income homebuyers to sign up for mortgages with a high risk of never getting paid back.
This continuous buildup of toxic assets, eventually caused the bursting of the US housing bubble, creating a perfect storm for the deepest recession within and beyond US borders, since 1929.
The main lesson from the 2008 Global Financial Crisis is that no nation, company or consumer is immune to the effects of shocks from neighbouring countries and other economic agents in their own economies.
While the effects of transitory shocks are normally short lived, permanent shocks can impact a firm’s immediate and future productivity plus cash flows1.
The goal of macroprudential polices is to increase the financial system's resilience to shocks, by identifying and addressing systemic risks that cause severe loses across the financial system.
Firms deal with businesses to produce goods and services, banks lend to each other and nations trade to facilitate growth, creating links and wider networks.
Financial networks are dynamic and vulnerable to shocks, as these can generate new links and strengthen existing ones, with varying degrees of persistence.
The effects of shocks can move through the economy in uncertain ways, which highlights the importance of understanding how networks facilitate this process in the short and long-term.
Identifying risk by monitoring persistence in uncertainty networks
In their joint investigation, Michael and colleague Professor Jozef Barunik from Charles University, develop a novel approach to track how networks change over time in response to temporary and persistent shocks.
An analysis2 of 2005-2018 stock return volatility data from S&P500 American firms across 11 sectors3, reveals risk from different industries spills over to one another and long-term connections intensify in conjunction with market downturns.
In other words, the model suggests systemic risk stems from persistent network structures4.
Using daily data, the framework visually illustrates the direction and strength of a sector’s network connections caused by transitory and persistent shocks on a particular day.
By highlighting firms that send more shocks than they receive, it offers a quantitative measure of how individual institutions influence systemic risk, which helps identify systemically important financial institutions (SIFIs)5.
A new measure to identify uncertainty hubs and predict business cycles
One of the framework’s main advantages is its applicability to a wide range of financial phenomena6.
Mattia, Jozef and Professor Robert Faff from University of Queensland, follow a similar approach to develop a new measure of network uncertainty from US industries that helps predict future business cycles up to one year ahead. The new measure indicates the existence of “uncertainty hubs”, which are industries with the potential to spread uncertainty to other sectors and the real economy. Instead of using stock prices, they use changes in option prices to study networks that form on shocks to investors’ expectations, or "fears"7.
Using 2000-2020 option price data for a large sample of US firms, they developed a predictive network uncertainty index which considers investors’ future expectations on the next month’s industry uncertainty.
The analysis reveals communications, IT, finance, and real estate sectors as those with the highest contributions to overall network uncertainty and, consequently, the ones with the strongest effect on the business cycle.
This means prices, production, employment and growth were not only impacted in these sectors, but there were also uncertainty spillovers to other industries, ultimately affecting the broader economy.
Benefits for central banks and investors
Both methods provide precise point-in-time estimates useful to analyse network connectedness daily, monthly or in relation to a business cycle period of interest.
On a macroeconomic level, these monitoring tools offer valuable insights to refine policies aimed at mitigating adverse firm behaviour.
For example, macroprudential policy makers and analysts may use it to provide a ranking on how risky financial firms are at a specific time, which helps assess whether a sector of SIFI influence is temporary or long lasting.
This could act as a signal to monitor balance sheets or lending positions and allow policy responses at bank level (eg increasing capital requirements for firms/sectors that contribute significantly to systemic risks).
Changes in networks derived from uncertainty hubs can serve as leading indicators of the business cycle, which means policy interventions can be targeted at mitigating the impact of their fluctuations.
Specifically, reducing shocks in sectors like financials, communications and IT could prevent excessive uncertainty propagation, which can potentially lead to business cycle contractions.
Central banks with an inflation targeting mandate may use it to understand how long an interest rate change is needed to counter inflation without triggering an economic slowdown.
On a microeconomic level, financial institutions could use the models to track the riskiness of other banks or firms applying for loans, and hence influence their lending decisions.
1 Temporary shocks can be caused by, for example temporary changes in demand or supply chain disruptions, but do not have a long-term impact in the company’s productivity and cash flows. In contrast, permanent shocks caused by disruptive technologies, trade barriers, etc. cause longstanding effects on the business.
2 Network connectedness is measured using frequency domain techniques, specifically time-varying spectral decompositions.
3 11 main sectors according to the Global Industry Classification standard: Consumer Discretionary, Consumer Staples, Health Care, Industrials, Information Technology, Materials, Real State, Financials, Energy, Communication Services and Utilities.
4 The model shows spikes in persistent network connectedness when long-lasting financial and economic events occur.
5 See also previous work by Mattia on the forward-looking measures of the network connectedness of fears in the financial system.
6 Current projects are implementing a similar approach in the study of price network risk in currency markets, climate change impact on financial markets and sectoral connections in Japanese and Chinese financial markets
7 Options contracts give investors the right to buy or sell a financial asset at a predetermined price before a specified day, therefore price fluctuations provide a reliable picture of the future risk and investors’ expectations about the performance of an industry
![]() |
Senior Lecturer in Finance |
![]() |
Lecturer in Finance |
You can find out more about Michael and Mattia's work here: Ellington, M. (2022). 'Fat tails, serial dependence, and implied volatility index connections', European Journal of Operational Research, 299(2), 768–779. BaruníkBevilacquaTunaru, R. (2022). 'Asymmetric Network Connectedness of Fears', The Review of Economics and Statistics, 104(6), 1304–1316. Baruník Ellington, M. (2024). 'Persistence in financial connectedness and systemic risk', Journal of Economic Dynamics and Control, 159. BaruníkBevilacqua, R. (2024). 'Asymmetric Network Connectedness of Fears',The Review of Economics and Statistics, 104(6), 1304–1316. |
Keywords: Accounting and Finance, Systemic risk, Financial uncertainty.