New Deep Learning Model Improves Prostate Cancer Diagnosis by Enhancing MRI Segmentation
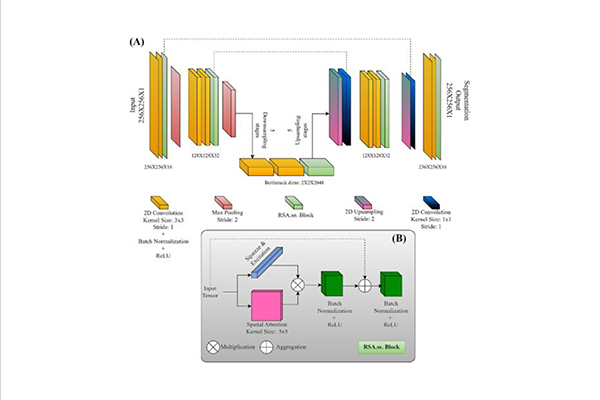
A new deep learning (DL) model called ResQu-Net has demonstrated significant improvements in the segmentation of the peripheral zone of the prostate, a critical region for the diagnosis and treatment of prostate cancer. Prostate cancer, which is one of the leading causes of cancer in men, often arises in the peripheral zone, making accurate delineation of this area on MRI scans crucial for effective clinical decision making.
The peripheral zone is notoriously difficult to segment accurately due to its complex anatomical structure, and until now, no DL algorithms have been specifically tailored to this task. The ResQu-Net model, however, has been designed to address these challenges and deliver more precise results. In a recent study, a team of researchers including former OMA Fellow Charalampos Kalantzopoulos tested ResQu-Net on three publicly available datasets and compared it to six other popular DL segmentation models, including Attention U-Net, Dense2U-Net, and U-Net. The results were compelling: ResQu Net outperformed the other models in accurately segmenting the peripheral zone across various anatomical regions, including the apex, midgland and base of the prostate.
Quantitative assessments showed that ResQu-Net improved segmentation performance by more than 5% in terms of Dice Score (a measure of overlap between predicted and true boundaries) and reduced the 95% Hausdorff Distance (a measure of the greatest distance between predicted and true boundaries) by 1.87 mm, compared to the other models. Qualitative evaluation, including analysis of feature maps, also demonstrated ResQu- Net's superior ability to match the ground truth annotations with greater precision.
These findings suggest that ResQu-Net could play a key role in addressing one of the major challenges in prostate cancer imaging, leading to better clinical outcomes by improving the accuracy of detection and treatment planning. The model’s ability to provide more accurate and reliable segmentation of the peripheral zone could significantly aid radiologists and clinicians in making more informed decisions for patient care.
Image:
ResQu-Net model (Fig. A) consists of an encoder decoder network which assists on the forward passing of information, being capable of retaining the spatial information of the prostatic zone by utilizing residual connections. The novel RSA.se block (Fig. B) involves the parallel connection of Squeeze and Excitation (SE) and Spatial Attention (SA) layers, along with the input’s residual connection, to propagate information smoothly.
Full article:
Zaridis, DimitriosI I.; Mylona, Eugenia; Tachos, Nikolaos; Kalantzopoulos, Charalampos N.; Marias, Kostas; Tsiknakis, Manolis; Matsopoulos, George K.; Koutsouris, Dimitrios D.; Fotiadis, Dimitrios I., “ResQu-Net: Effective prostate's peripheral zone segmentation leveraging the representational power of attention-based mechanisms”, BIOMEDICAL SIGNAL PROCESSING AND CONTROL 93, 106187 (JUL 2024)