Machine Learning Sheds Light on the Milky Way's History
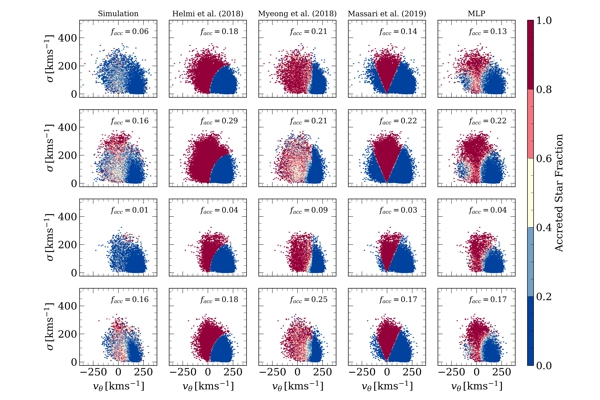
LIV.INNO student Andrea Sante has recently had a paper, ‘Applying machine learning to Galactic Archaeology: how well can we recover the origin of stars in Milky Way-like galaxies?’ published in the Monthly Notices of the Royal Astronomical Society. This paper discusses a new technique using machine learning to find stars that live in our galaxy but were born in other, smaller, galaxies which got cannibalised by the Milky Way.
This research has the potential to contribute significantly to our understanding of the formation of our galaxy and others alike.
Traditionally, astronomers have relied on simple distinctions in stellar motions, chemical makeup, and position to determine if a star formed within the Milky Way (in-situ) or originated in a smaller galaxy that was later devoured by our own (accreted). However, this method is not always effective, especially when looking at densely populated areas like the galactic disc.
The new approach utilizes machine learning algorithms trained on data from complex computer simulations of galaxy formation. These algorithms can combine in complex ways all the available information on a star to determine its birthplace with greater accuracy.
"The models can identify accreted stars even in regions heavily dominated by in-situ stars," said lead author Andrea Sante. This is a significant improvement, as it allows scientists to peer into the crowded disc and identify stars that may hold clues to the Milky Way's past mergers.
The technique was tested on additional simulations, demonstrating its effectiveness on unseen data. This bodes well for applying the method to real-world observations, potentially revealing previously hidden stellar streams and substructures within the Milky Way.
By deciphering the origin stories of our galaxy's stellar inhabitants, astronomers can piece together a more complete picture of galactic formation and evolution. This new machine learning technique offers a powerful tool to unlock the secrets written in the stars.
More information:
‘Applying machine learning to Galactic Archaeology: how well can we recover the origin of stars in Milky Way-like galaxies?’, Andrea Sante, Andreea S Font, Sandra Ortega-Martorell, Ivan Olier, Ian G McCarthy, Monthly Notices of the Royal Astronomical Society, Volume 531, Issue 4, July 2024, Pages 4363–4382
https://doi.org/10.1093/mnras/stae1398
* Image: Comparison of the distribution of accreted (red) and in-situ (blue) stars colour-coded in the simulated Solar neighbourhoods. The rows corresponds to four different simulated galaxies. In the columns, the accreted stars are defined by: 1) the simulation label; 2-4) the observational selection criteria; 5) the label predicted by the MLP model, developed in this study. (Image credit: A. Sante, et al. Monthly Notices of the Royal Astronomical Society, Volume 531, Issue 4, CC-BY)