Using AI to leverage new forms of data in modelling cycling behaviours in the LCR
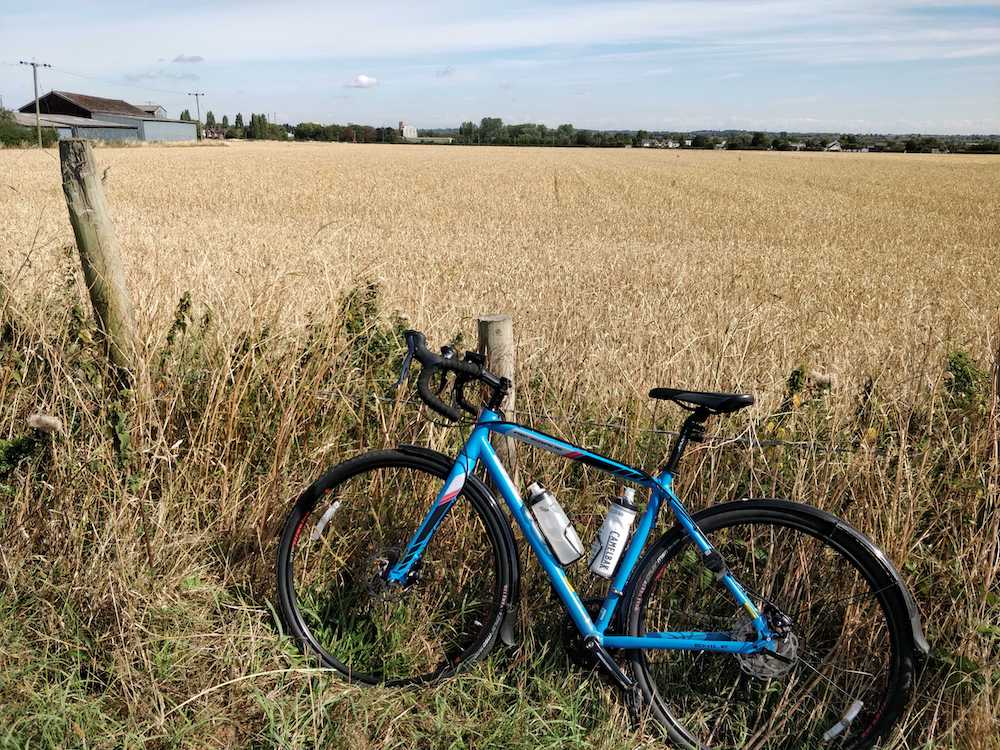
We are excited to announce a new PhD post supervised by Dr. Mark Green (mgreen@liverpool.ac.uk, Geography & Planning), Dr. Dani Arribas-Bel (d.arribas-bel@liverpool.ac.uk, Geography & Planning), and Dr. Shagufta Scanlon (Shagufta.Scanlon@liverpool.ac.uk, Computer Science), in partnership with the Liverpool City Region.
The Problem
It is estimated that 22% of adults in England are physically inactive, and these rates are higher within each Local Authority within the Liverpool City Region (LCR) (PHE 2019). Physical activity is an important determinant of health, being associated with lower risk of cardiovascular diseases, as well as improved mental wellbeing (Warburton and Bredin, 2017). Designing cities and neighbourhoods to encourage physical activity is therefore an important policy priority. An increasingly adopted approach is to increase the uptake of active travel, particularly cycling (DfT 2017). However, only 3.3% of adults in England cycle for travel at least 3 times per week, and rates are lower for the LCR (PHE 2019). Targeted investment in cycling infrastructure can encourage more individuals to take up cycling (Hull and O’Holleran, 2014), as well as reduce air pollution indirectly benefiting health.
The Solution
This project will use state-of-the-art machine learning and AI techniques to leverage new forms of data to improve decision making around cycling investment. Such approaches are rarely applied within transport modelling (Hagenauer and Helbich, 2017), but offer novelty to process and model complex (big) data to inform cycling behaviours and infrastructure provision. A key advantage of the PhD will be the development of bespoke methods that enable to make the most out of data unexplored in the context of cycling. These methods will be designed so that they can be easily deployed within any local government to inform cycling provision. This project is designed to co-produce real-world solutions alongside the non-academic partner, the LCR, thus maximising impact.
Outline of the PhD
The project will be structured as a publication-based PhD, and will include three main
subprojects, which will be complemented with introduction and conclusion sections. The three core chapters will be:
- Modeling volume of cycling traffic from pneumatic road tube counters
This project will use cycling counts from pneumatic road tube counters and ancillary data about the characteristics of the locations where they are placed to build a predictive model of cycling counts at the street segment that can be deployed to the entire network of the LCR. This will enhance the understanding of the distribution of cyclists to agencies related a range of domains, from public health to transport planning. Methodologically, this project will expand tree-based models (e.g. random forests, boosted trees) to explicitly incorporate spatial features and relationships.
- Understanding the drivers behind cycling flows
This paper will unpack the driving factors behind the estimates obtained in the previous one. By combining traditional socio-economic sources of data (e.g. Census, Deprivation scores) with new approaches such as video footage or imagery data that recognise features of the environment (e.g. road quality, foliage, etc.), the study will identify how environmental factors interact with social conditions to determine the extent to which people cycle in different places. To be able to leverage these data sources, state-of-the-art AI techniques such as convolutional neural networks (CNNs) will be required.
- Predicting where to invest on urban cycling infrastructure
In this final paper, the student will use results from the previous two in order to build a decision-making system that informs policies on improvement of cycling infrastructure in the LCR. The system will fulfill two main functions: first, it will provide an intuitive way of visualising and interacting with the results of the predictive models generated and the measures of uncertainty associated with them; second, it will feature the capability of asking “what-if” type of questions around the improvement of infrastructure. In this context, the student will explore the suitability of spatial interaction and agent-based models. It is expected this system will enable the identification of policy priorities within the LCR.
Data Sources
- Bike traffic volume bands (provided by the LCR)
- Aerial/satellite imagery
- Imagery/footage of cycling routes (potentially linked to current project in Planning)
- Socio-economic characteristics (Census, etc.)
- Street network (OS roads)
Background and contact information
The project is supported by the University of Liverpool Doctoral Network in Future Digital Health, which is directed at creating and maintaining a community of AI health care professionals that can realise the benefits that AI can bring to Health Care. The vision is that of a world-class centre providing high-quality doctoral training within the domain of AI for Future Digital Health. Each available PhD project has been carefully co-created in collaboration with a health provider and/or a healthcare commercial interest so that the outcomes of the PhD research will be of immediate benefit. The network will be providing doctoral training, culminating in a PhD, in a collaborative environment that features, amongst other things, peer-to-peer and cohort-to-cohort based learning. On completion students will be well-placed to take up rewarding careers within the domain of AI and Digital Health.
Deadline: 28 May 2019
Funding: This project is funded by the University of Liverpool Doctoral Network in Future Digital Health, successful students will receive a studentship of tuition fees paid at the Home/EU rate for 3.5 years and a stipend of £15,009 per annum for 3.5 years. In addition, students will have access to a research support fund of £1,000 per annum for purchasing equipment, consumables and conference costs co-managed by the academic supervisor. Applications from international students are welcomed, however suitable arrangements will need to be made for the difference between the Home/EU and international rate.
Apply here: https://www.liverpool.ac.uk/study/postgraduate-research/how-to-apply/
References
Department for Transport (DfT), 2017, Cycling and Walking Investment Strategy, https://assets.publishing.service.gov.uk/government/uploads/system/uploads/attachment_data/file/603527/cycling-walking-investment-strategy.pdf(last accessed 7th March 2019).
Hagenauer J, Helbich M, 2017, A Comparative Study of Machine Learning Classifiers for Modeling Travel Mode Choice, Expert Systems with Applications78: 273–82.
Hull A, O’Holleran C, 2014, Bicycle infrastructure: can good design encourage cycling?, Urban, Planning and Transport Research2: 369-406.
Public Health England (PHE), 2019, Public Health Profiles: Physical Activity, https://fingertips.phe.org.uk/profile/physical-activity(last accessed 7th March 2019).
Warburton D, Bredin S, 2017, Health benefits of physical activity: a systematic review of current systematic reviews,Current Opinion in Cardiology32:541–556.