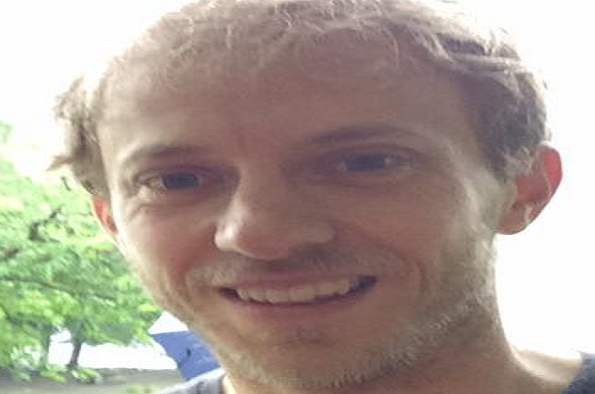
Deciphering Complex Metabolite Mixtures by Unsupervised and Supervised Substructure Discovery and Semi-Automated Annotation from MS/MS Spectra
- Andy Jones
- Suitable for: Those interested in Genomes, Systems and Therapeutic Targeting
- Admission: Free
Add this event to my calendar
Click on "Create a calendar file" and your browser will download a .ics file for this event.
Microsoft Outlook: Download the file, double-click it to open it in Outlook, then click on "Save & Close" to save it to your calendar. If that doesn't work go into Outlook, click on the File tab, then on Open & Export, then Open Calendar. Select your .ics file then click on "Save & Close".
Google Calendar: download the file, then go into your calendar. On the left where it says "Other calendars" click on the arrow icon and then click on Import calendar. Click on Browse and select the .ics file, then click on Import.
Apple Calendar: The file may open automatically with an option to save it to your calendar. If not, download the file, then you can either drag it to Calendar or import the file by going to File >Import > Import and choosing the .ics file.
Mass spectrometry (MS) is a widely used technique to obtain structural information on complex mixtures. We recently proposed a substructure-based strategy to enhance metabolite annotation for complex mixtures by considering metabolites as the sum of (bio)chemically relevant moieties that we can detect through mass spectrometry fragmentation approaches. Our MS2LDA tool allows us to discover - unsupervised - groups of mass fragments and/or neutral losses termed Mass2Motifs that often correspond to substructures. A drawback is that Mass2Motifs have to be manually annotated. I will describe how additional strategies, taking advantage of i) combinatorial in-silico matching of experimental mass features to substructures of candidate molecules, and ii) automated machine learning classification of molecules, can facilitate semi-automated annotation of substructures, and enhance structural characterisation of metabolites.