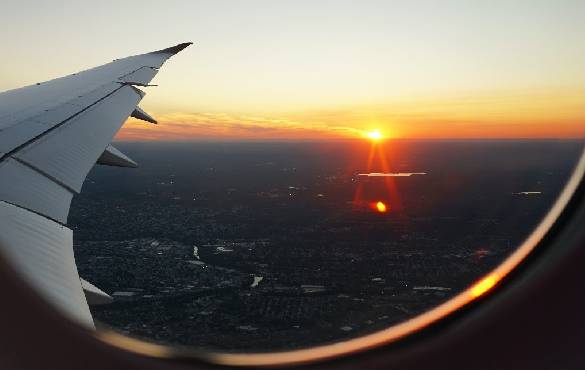
Mehdi Anhichem, Year 4 PhD student at the CDT presents his latest research at the AIAA Scitech Forum in Orlando on January 8, 2024
Background
Understanding how airplanes fly and designing them efficiently is crucial. Engineers rely on computational fluid dynamics, a resource-intensive simulation method, to understand the impact of air on aircraft shapes. In my recent research, I delve into the application of machine learning, specifically Bayesian models, to construct surrogate models that approximate crucial information for aircraft design. These models not only make predictions but also provide insights into their confidence levels. The study aims to evaluate the performance of these models in predicting air pressures on aircraft surfaces, considering both accuracy and the models' confidence in their predictions.
Importance of the research
This research is motivated by the growing interest within the aerodynamic community to harness cutting-edge machine learning techniques, particularly in the context of surrogate modelling. Surrogate modelling holds significant importance in engineering design across various fields, where experimental or numerical methods are employed to infer quantities of interest. However, the challenge arises in quickly and comprehensively exploring the design space, prompting the use of surrogate models to approximate experiments. Recent advancements in machine learning present innovative solutions for enhancing surrogate modelling applications.
In aerodynamics, surrogate modelling proves invaluable for approximating scalar quantities like integrated forces and moments, as well as distributed quantities such as pressure distributions on wings under different flight conditions. The prohibitive costs associated with using computational fluid dynamics (CFD) or wind tunnel experiments for tasks requiring numerous evaluations, like shape optimization or uncertainty quantification, underscore the need for efficient surrogate models. Gaussian process regression and neural networks have been primary choices in the literature. This research focuses on predicting pressure distributions on a wing's surface amid varying flight conditions. The primary challenges lie in handling the substantial amount of data generated from CFD simulations and accounting for the diverse flow characteristics associated with different flight conditions.
Given that surrogate models are inherently approximations, the emphasis here is on the quality of their predictions, particularly in the context of aerospace design, where there is virtually no margin for error. The interest here is the epistemic uncertainty associated with the surrogate model with respect to its input data. Bayesian methods offer a robust framework for estimating epistemic uncertainty in surrogate models. In this study, two Bayesian models, Gaussian processes, and Bayesian neural networks, are explored. The research findings will be presented at the AIAA Scitech Forum in Orlando on January 8, 2024, with corresponding proceedings to follow shortly.
What comes next?
Given the promising outcomes of the current study, our next step involves applying these findings to a more complex geometry representative of a commercial aircraft. This expanded investigation aims to assess the performance of Bayesian surrogate models under more complex conditions. Additionally, we will delve deeper into the uncertainty calibration of these models to enhance their reliability in predicting aerodynamic pressure distributions.