Research News: Launch of Stone Soup Version 1.0
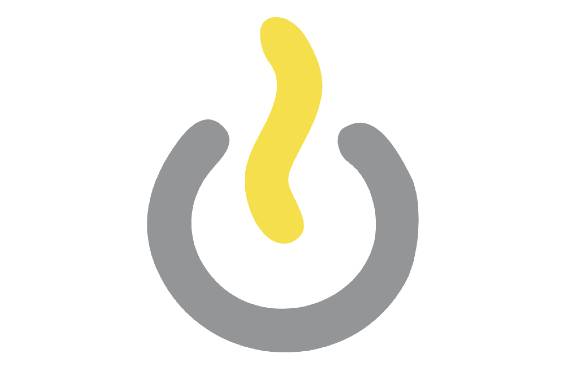
Benedict Oakes and Lyudmil Vladimirov provide an introduction to their paper, introducing Stone Soup 1.0, which will be presented at this year's Fusion Conference 2023.
Summary
In this paper, we announce the launch of Stone Soup version 1.0. Stone Soup is an open-source Python framework for tracking and state estimation maintained by Dstl, and supported by a wide community of open-source code contributors. Stone Soup is designed as a modular framework with easily configurable components that can be arranged in a number of ways to build and compare target tracking and data fusion algorithms. Stone Soup has been in development in the form of a beta version for many years, and has now reached a stable point that contains many algorithms and use cases.
Importance of the research
Stone Soup was originally conceived from the difficulty that researchers in the state estimation field have in being able to benchmark novel tracking algorithms against the state-of-the-art. Stone Soup contains many algorithms that novel work can be benchmarked against, and a framework that allows users to easily add their own algorithms to in a modular way, that allows it to be tested in a range of scenarios and use-cases. This allows a rigorous comparison between different algorithms, so that the best algorithm can be chosen for any given scenario. Stone Soup contains models for scenarios including space, air, ground, and maritime for tracking applications.
What comes next?
Although this marks the release of version 1.0, development continues to add new features and algorithms. Further work is likely to include but not limited to, multiple hypothesis tracking, track before detect, reinforcement learning sensor management, and more. We aim for Stone Soup to become adopted widely throughout the state estimation and sensor fusion communities, both for use of testing algorithms, but also for contributions of new datasets, algorithms, models, or metrics.
This paper will be published as part of the Fusion 2023 Conference.