Research News: Multifidelity Data Fusion Applied to Aircraft Wing Pressure Distribution
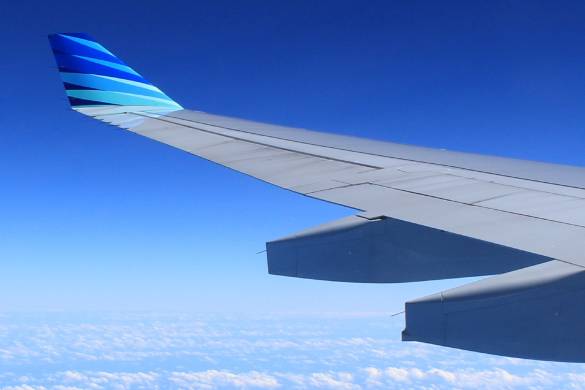
Mehdi Anhichem, PhD student at the CDT, provides an overview of his research paper 'Multifidelity Data Fusion Applied to Aircraft Wing Pressure Distribution'. which he'll present at AIAA Aviation Forum, 27 June - 1 July 2022, Chicago, Illinois.
Summary
Designing an aircraft requires studying its aerodynamics, i.e. the way its shape moves through air. The principal ways of acquiring such aerodynamic data are wind tunnel testing and numerical analysis. In wind tunnel experiments, a scaled model is manufactured to collect data about the air flow around the model through measurements. Aerodynamic data can also be computed with deterministic numerical simulations such as using an incarnation of the Navier–Stokes equations. They both can be expensive and are subject to multiple sources of uncertainty. Thus, there is a significant challenge to leverage information from the different aerodynamic data sources while controlling the definition and propagation of uncertainty towards the decision-making level.
Importance of the research
It may be debated within the aeronautics community that the digital revolution, especially through the introduction of numerical methods, such as Computational Fluid Dynamics (CFD), has not had the expected impact on wind tunnel usage for flight vehicle development programmes. In practice, CFD has been integrated into the industrial testing routine to complement the theoretical, numerical and experimental methods already in use. Nevertheless, digital engineering can be considered as an opportunity to rethink current practices and processes. The idea behind this work is to be able to combine different data sources in order to obtain a better overall estimate on the design space. Machine learning offers practical and efficient methods for merging the information from different data sets and for incorporating real world variability and probabilistic behaviour into engineering analysis. Uncertainty quantification takes place when making high-consequence decisions within the risk management step of the design cycle. Thus, the ambition of this work is to advance aerodynamic design of future aircraft through the application of machine learning algorithms that enable experimental and numerical data to be combined while accounting for uncertainty.
What comes next?
The next steps of my work will be to study the machine learning algorithms previously applied in this paper and to improve them with respect to the data involved in commercial aircraft analysis and design. I will also look at defining more accurately the physical uncertainty of the different information sources over the design space. The objectives are to improve surrogate modelling based on aerodynamic data and to incorporate the expert opinion and confidence into the data fusion approach.