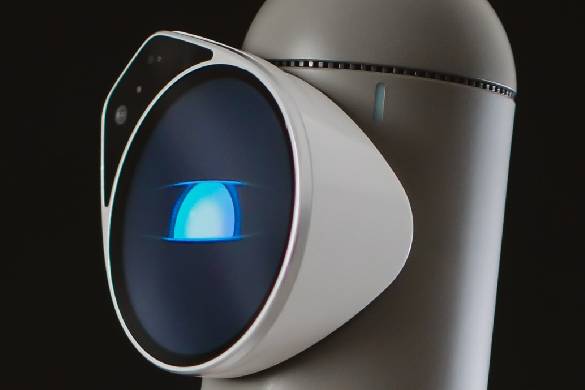
Elinor Davies, PhD student at the CDT, provides an overview of her research paper 'A multi-Bernoulli Gaussian filter for track-before-detect with superpositional sensors'
Summary
Multiple target tracking is an important area of research with many applications. When there is a low signal-to-noise ratio Track-before-detect methods have been shown to be more accurate than popular detection based methods. This is because raw measurement data is used which prevents the loss of potentially useful tracking data. I propose a Gaussian implementation of a multi-Bernoulli Track-before-detect filter for multi-target tracking with superpositional sensors. In this implementation a Bernoulli filter for each potential target is run independently. Bernoulli filters share their predicted measurement information with each other allowing them to account for the influence of other targets.
Importance of the research
We were interested in track-before-detect methods and their application to a superpositional sensor model. We were also interested in developing an algorithm that would allow for a parallel implementation. We therefore focused on an Independent Multi-Bernoulli (IMB) filter where each potential target is tracked by an independent Bernoulli filter. Here parallel computing is applicable because different Bernoulli filters could run in parallel. Unfortunately, the existing method does not allow for the use of superpositional sensors. Therefore, we proposed the Information Exchange Multi-Bernoulli (IEMB) filter, a modification to the existing method that could work with superpositional sensors. The filters were tested on their performance when using superpositional sensors and the IEMB filter performed considerably better than the IMB filter. Therefore the IEMB filter is more applicable to scenarios where targets may be in close proximity and sensors can observe more than one target at a time.
What comes next?
Future work includes the parallelisation of the filter, the implementation of different filtering methods, such as particle filters, or the iterated posterior linearisation Filter. Another line of future work is to improve the performance when moving targets are in close proximity. We hope to extend the current work and work towards a journal paper. We are also interested in applying the filter to real sensor data provided by industrial partner Leonardo.
This article belongs to the CDT's Fusion 2022 series. Please review our other Fusion conference paper overviews.