Research News: Double Deep Q Networks for Sensor Management in Space Situational Awareness
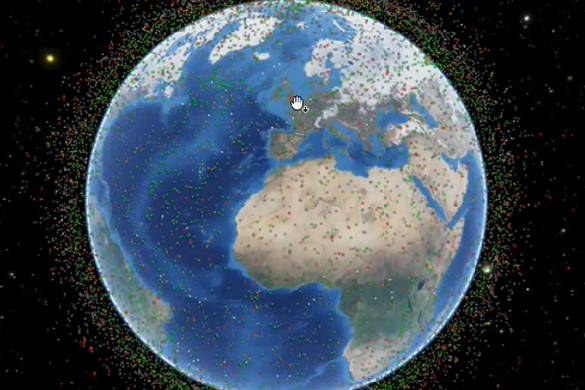
Benedict Oakes, PhD student at the Distributed Algorithms CDT, introduces his latest research paper.
Summary
We have created a novel application of a reinforcement learning environment to a space situational awareness scenario. There are increasing numbers of satellite being launched into orbit, but only a limited number of sensors available. We aim to use reinforcement learning to utilise these limited sensor resources to observe and track more satellites reliably.
Importance of the research
The space domain has become a more crowded environment in recent years, with an increasing number of satellite launches, such as the Starlink satellite constellations. We use these satellites in everyday life, for communication, science, GPS, defence, and others. With an increasing number of satellites comes an increasing risk. Satellite collisions that produce significant debris could cause a cascading effect known as Kessler syndrome, where each piece of debris will collide with more satellites and produce more debris, rendering certain orbits unusable. To attempt to mitigate this risk, we must first understand the space domain. We can use ground-based telescopes to make observations of satellites, and to track their trajectories. This becomes more difficult as the number of satellites increase. We hope to use reinforcement learning for sensor management, to increase the number and quality of satellite trajectories we are able to follow. In this paper, we present a novel application of a reinforcement learning agent to this problem. The agent learns to track satellites, and reduce their position and velocity uncertainties. This paper shows that reinforcement learning can be applied to this problem, and shows some promising initial results. Reinforcement learning allows us to simplify complicated environments into more manageable problems, which is useful in this area due to the large number of satellites.
What comes next
This paper acts as a baseline for future work. We aim to expand our environment to include more complicated aspects, and to more accurately model the telescope and satellites. Going forward, we aim to use reinforcement learning to tackle the non-myopic (long-sighted) problem with space situational awareness; for example, being able to predict and observe future collision events. This would allow us to track the resulting debris, and to use this to prevent further collisions.
Visit the link to the full paper here.
Authors: Benedict Oakes, Dominic Richards, Jordi Barr, Jason F. Ralph
This article belongs to the CDT's Fusion 2022 series. Please review our other Fusion conference paper overviews.