Research News: One step closer to success – proving efficiencies with No-U-Turn Sampler techniques
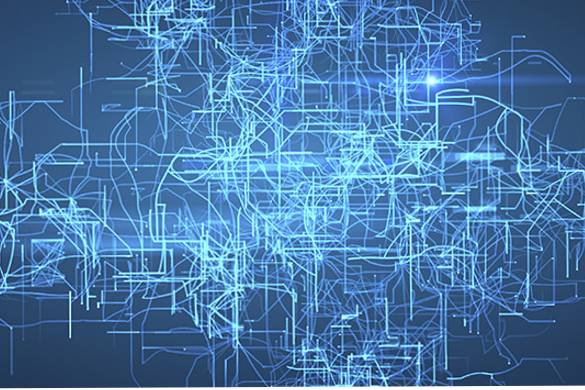
Conor Rosato, post doc researcher at the Signal Processing Group, shares his thoughts on his latest research work.
The research
We have devised a way to differentiate a particle filter which allows us to use gradient based proposals within particle- Markov Chain Monte Carlo (p-MCMC). It has been widely documented that the sampling and resampling steps in particle filters cannot be differentiated. The reparameterisation-trick was introduced to allow the sampling step to be reformulated into a differentiable function. We extend the reparameterisation-trick to include the stochastic input to resampling therefore limiting the discontinuities in the gradient calculation after this step. Knowing the gradients of the prior and likelihood allows us to run p-MCMC and use the No-U-Turn Sampler (NUTS) as the proposal when estimating parameters.
Why it matters
The benefits of using p-MCMC over Markov Chain Monte Carlo (MCMC) methods can be seen when performing Bayesian inference in non-linear non-Gaussian scenarios when the posterior evolves over time and more data becomes available. Current research uses random-walk proposals such as Metropolis-Hastings (MH) and Gibbs techniques which can struggle to reach the stationary distribution when estimating large amounts of parameters. It is anticipated that gradient based methods will cut the computation time and improve the mixing of Markov chains at the stationary phase and decrease the length of burn-in.
We’re thinking
That we can move away from using random-walk proposals such as Metropolis-Hastings (MH) and Gibbs techniques and start using Hamiltonian Monte Carlo (HMC) based methods. In the near future a published paper will outline the methods for general state-space models, with our focus shifting to epidemiological models and COVID-19. Work is also underway to make this research accessible to others by incorporating the ideas into Streaming-Stan which is a probabilistic programming language.
Conor Rosato, Electrical Engineering and Electronics, University of Liverpool